by Yara Ghazal, Ilyana Hohenkirk, Tracy Kugler, and Kelly Searle
Malaria, like many vector-borne diseases, impacts health, economic growth, and society. The burden of malaria incidence and death is concentrated in Sub-Saharan Africa; in 2020, 95% of all malaria cases and 96% of all deaths occurred in Sub-Saharan Africa (WHO, 2022). Malaria impacts not only population health but also the economic growth of these 32 countries. It is estimated that up to 1.3% of economic growth in this region of Africa is slowed each year due to malaria (CCP-JHU, 2015). Understanding malaria transmission is essential to ending its spread and creating a healthier and more prosperous future for developing nations.
The literature on malaria transmission patterns has shown that several environmental factors impact mosquito and parasite vital rates, and thus affect the transmission intensity, seasonality, and geographical distribution of malaria (Castro, 2017). Temperature and precipitation are the primary climate-based factors that influence malaria transmission patterns. Temperature creates geographical constraints for vector and parasite development. Increasing temperatures have been found to shorten mosquito maturation time and increase feeding frequency. However, areas of extremely high temperatures usually yield smaller, less fecund mosquitoes. In parallel, because mosquitoes often breed in pools formed by rainfall and flooding, the frequency, duration, and intensity of precipitation have a significant influence on mosquito populations.
Studies have also found that malaria risk is influenced by demographic factors such as race and gender, with the highest malaria prevalence rates being among females and children (Gunathilaka et al., 2016). Urbanization, agriculture, and infrastructure have also been identified as factors affecting malaria risk (Castro, 2017).
During our 2022 Summer Diversity Fellowship, we created a centralized database combining population, ecological, and agricultural data to enable the study of spatial patterns and interaction of various variables in relation to malaria transmission and its impact. We completed the data linking process for Angola and Mozambique, and conducted initial data work for Gambia, Nigeria, Ghana, and Togo.
Our data sources and the types of variables we extracted from them included:
- The Demographic Health Survey (DHS)
- Survey data on household characteristics, water sources, and land use
- Environmental variables linked by the DHS program, including precipitation, vegetation, humidity, aridity, and average temperatures
- The Malaria Indicator Survey (MIS, a supplemental module to the DHS)
- Malaria-specific data, such as the use of Artemisinin combination therapy, malaria rapid testing, and mosquito net use and treatment
- IPUMS International
- Demographic records, educational attainment, and additional urbanization data
- IPUMS IHGIS (International Historical Geographic Information System)
- Agricultural census data on agricultural land ownership, agricultural workforce, livestock, and crops.
In order to connect data across these data sources, we used geographic linkages. DHS and MIS data are associated with “cluster points” representing the approximate centroids of villages or other areas in which survey respondents live. IPUMS International and IHGIS data were aggregated in geographic units such as districts. To make the linkages, we obtained shapefiles describing the locations of cluster points and boundaries of IPUMS International and IHGIS units.
The data-linking process consists of two major steps, geographic linkages and table joins. We used GIS software (QGIS) to perform the geographic join between cluster points and geographic units. QGIS leverages the latitude/longitude of each cluster point to determine the geographic unit where the point was located; we then used QGIS to produce a table connecting each cluster ID to a geographic unit ID (Fig. 1). For each country, we typically had two sets of geographic units (e.g., states and counties). We performed geographic joins for each set, locating clusters within the first- and second-level administrative units.
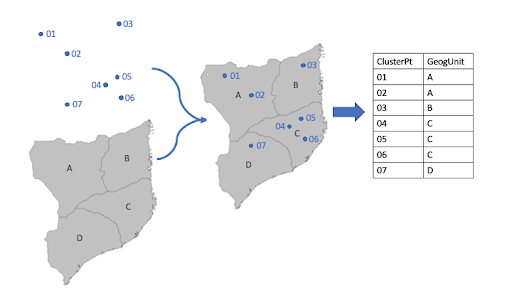
We then used the linked cluster and geographic unit IDs to attach contextual variables measured at the cluster-level and at the geographic-unit-level from multiple data sources. Contextual variables from DHS and MIS were measured around cluster points and were joined based on the cluster ID. Contextual variables from IPUMS International and IHGIS were measured within geographic units and were joined based on geographic unit IDs. These table joins were conducted in R.
The final product consists of records from the MIS augmented with contextual variables from the DHS, IPUMS International, and IPUMS IHGIS. In addition to the six countries for which we have started linking work, we hope to complete this process for 15 additional African countries as more data become available. Having a single master file of linked data will enable researchers to efficiently incorporate demographic information, health data, and contextual variables in analyses to shed light on the factors that affect the spread of malaria.
This work was supported by the National Science Foundation, award 2121891.
References
Castro, M. (2017). Malaria transmission and prospects for malaria eradication: The role of the environment. Cold Spring Harbor Perspectives in Medicine, 7(10), A025601.
Gunathilaka, N., Abeyewickreme, W., Hapugoda, M., & Wickremasinghe, R. (2016). Determination of demographic, epidemiological, and socio-economic determinants and their potential impact on malaria transmission in Mannar and Trincomalee districts of Sri Lanka. Malaria Journal, 15(1), 330.
Johns Hopkins School of Public Health Center of Communications Program. (2015). Malaria. Malaria Free Future. https://www.malariafreefuture.org/malaria#economic_impact
World Health Organization. (2022). World malaria report 2022. https://cdn.who.int/media/docs/default-source/malaria/world-malaria-reports/world-malaria-report-2022.pdf?sfvrsn=40bfc53a_4